Research Areas
I currently hold a professorship of Artificial Intelligence at the Carinthia University of Applied Sciences. My research focusses on the interface between machine learning and statistics, applied to solve real-world problems across domains. During my previous positions, my work was funded by ECSEL Joint Undertaking/Austrian Research Promotion Agency (project SemI40, 2016-2019) and by the Norwegian Cancer Society (project Bioradiance, 2019-2020).
Data science | machine learning | AI
probabilistic models for machine learning, un-/semi-supervised learning
Applied statistics
Bayesian statistics, computational statistics, design of experiments, spatial and temporal models
Interpretability and explainability in AI
feature selection and ranking, domain knowledge in machine learning, explainable AI
Healthcare applications
treatment selection and outcome prediction, feature extraction from medical images
Time series analysis
time series models for forecasting, classification and segmentation
Industrial applications
process monitoring, error detection via pattern recognition
Resume
Research Positions
Professor of Artificial Intelligence
2024 - present
Carinthia University of Applied Sciences, Villach, Austria
Artificial Intelligence expert
2024
Infineon Technologies Austria AG, Villach, Austria
- developed AI methods to improve the production processes and product quality in semiconductor manufacturing
- collaborated with academic partners in funding projects
Postdoctoral fellow in data science
2021-2023
Norwegian University of Life Sciences, Ås, Norway
- developed machine learning methods for time series analysis in life science use-cases
- conducted research on interpretable and explainable machine learning models, and the integration of expert knowledge in feature selection
Visiting researcher
March - June 2022
University of British Columbia, Vancouver, Canada
- conducted research in applied statistics for hydrological and ecological use-cases
- financed from internal funding scheme at Norwegian University of Life Sciences (project number 1211130114)
Postdoctoral fellow in data science
2019 - 2020
Oslo University Hospital, Oslo, Norway
- developed and applied machine learning for medical cancer imaging, microscopic imaging and treatment outcome prediction
Industrial Master's / PhD student
2015 - 2019
KAI GmbH (part of Infineon Technologies), Villach, Austria
- conducted research on data science methods for pattern recognition
- implemented machine learning in process control for semiconductor manufacturing
- investigated experimental designs for semiconductor lifetime studies
Education
Doctoral degree (PhD) in computer science
2016 - 2019
Graz University of Technology, Austria
passed with distinction
Diplom-Ingenieur (Master's degree) in technical mathematics
2014 - 2016
University of Klagenfurt, Austria
specialized in applied statistics
passed with distinction
Bachelor of Science in technical mathematics
2011 - 2014
University of Klagenfurt, Austria
specialized in applied statistics
passed with distinction
Scientific publications
Machine learning & statistics
- J. Janssen, S. Meng, A. Haris, S. Schrunner, J. Cao, W. J. Welch, N. Kunz, and A. A. Ameli: Learning from limited temporal data: Dynamically sparse historical functional linear models with applications to Earth science. Submitted, 2023. arXiv:2303.06501
- S. Schrunner, J. Janssen, A. Jenul, J. Cao, A. A. Ameli, and W. J. Welch: A Gaussian Sliding Windows Regression Model for Hydrological Inference. Journal of the Royal Statistical Society, Series C, 2025. doi:10.1093/jrsssc/qlaf009
- A. Jenul, H. L. Stokmo, S. Schrunner, M.-E. Revheim, G. O. Hjortland, and O. Tomic: Novel Ensemble Feature Selection Techniques Applied to High-Grade Gastroenteropancreatic Neuroendocrine Neoplasms for the Prediction of Survival. Computer Methods and Programs in Biomedicine, vol. 244, 107934, 2024. doi:10.1016/j.cmpb.2023.107934
- A. Jenul, S. Schrunner, B. N. Huynh, R. Helin, C. M. Futsæther, K. H. Liland, and O. Tomic: Ranking Feature-Block Importance in Artificial Multiblock Neural Networks. Artificial Neural Networks and Machine Learning -- ICANN 2022, 2022. doi:10.1007/978-3-031-15937-4_14
- A. Jenul*, S. Schrunner*, J. Pilz, and O. Tomic: A User-Guided Bayesian Framework for Ensemble Feature Selection (UBayFS). Machine Learning, 2022. doi:10.1007/s10994-022-06221-9
- A. Jenul, B. Bhattarai, K. H. Liland, L. Jiao, S. Schrunner, C. M. Futsæther, O.-C. Granmo, and O. Tomic: Component Based Pre-filtering of Noisy Data for Improved Tsetlin Machine Modelling. International Symposium on the Tsetlin Machine (ISTM), 2022. doi:10.1109/ISTM54910.2022.00019
- A. Jenul, S. Schrunner, K. H. Liland, U. G. Indahl, C. M. Futsæther, and O. Tomic: RENT - repeated elastic net technique for feature selection. IEEE Access, 2021. doi:10.1109/ACCESS.2021.3126429
- S. Schrunner, B. C. Geiger, A. Zernig, and R. Kern: A generative semi-supervised classifier for datasets with unknown classes, ACM Symposium on Applied Computing (SAC), 2020. doi:10.1145/3341105.3373890, Video
Semiconductor manufacturing
- S. Schrunner, M. Scheiber, A. Jenul, A. Zernig, A. Kästner, and R. Kern: Towards a General Framework to Embed Advanced Machine Learning in Process Control Systems. Submitted, 2022. arXiv:2103.13058
- S. Schrunner, A. Jenul, M. Scheiber, A. Zernig, A. Kästner, and R. Kern: A health factor for process patterns - enhancing semiconductor manufacturing by pattern recognition in analog wafermaps, IEEE International Conference on Systems, Man and Cybernetics (SMC), 2019. doi:10.1109/SMC.2019.8914309
- S. Schrunner, O. Pfeiler, A. Zernig, and J. Pilz: How to predict the lifetime of semiconductors in real-world applications with limited test resources?, in: Quality Management in Technology 2019, editors: J. Wittmann, W. Bergholz, indepedently published, 2019. Link
- S. Schrunner, O. Bluder, A. Zernig, A. Kästner, and R. Kern: A comparison of supervised approaches for process pattern recognition in analog semiconductor wafer test data, IEEE International Conference on Machine Learning and Applications (ICMLA), 2018. doi:10.1109/ICMLA.2018.00131
Image processing
- D. Arous, S. Schrunner, I. Hanson, N. J. Edin, and E. Malinen: Principal component-based watershed method for image segmentation of in vitro cancer cell colonies. Computer Methods in Biomechanics and Biomedical Engineering: Imaging & Visualization, 2022. doi:10.1080/21681163.2022.2035822
- M. Pleschberger*, S. Schrunner*, and J. Pilz: An explicit solution for image restoration using Markov Random Fields, Journal of Signal Processing Systems, vol. 92, pages 257-267, 2020. doi:10.1007/s11265-019-01470-9
- T. Santos*, S. Schrunner*, B. C. Geiger, O. Pfeiler, A. Zernig, A. Kästner, and R. Kern: Feature extraction from analog wafermaps: a comparison of classical image processing and a deep generative model, IEEE Transactions on Semiconductor Manufacturing, vol. 32 (2), pages 190-198, 2019. doi:10.1109/TSM.2019.2911061
- S. Schrunner, O. Bluder, A. Zernig, A. Kästner, and R. Kern: Markov random fields for pattern extraction in analog wafer test data, International Conference on Image Processing Theory, Tools and Applications (IPTA), 2017. doi:10.1109/IPTA.2017.8310124
Scientific software
- A. Jenul*, S. Schrunner*: UBayFS: An R Package for User Guided Feature Selection, Journal of Open Source Software, vol. 8 (81), 2023. doi:10.21105/joss.04848, CRAN
- A. Jenul, S. Schrunner, B. N. Huynh, and O. Tomic: RENT: A Python Package for Repeated Elastic Net Feature Selection, Journal of Open Source Software, vol. 6 (63), 2021. doi:10.21105/joss.03323
Academic theses
- S. Schrunner: Pattern recognition in analog wafer test data - a health factor for process patterns, PhD dissertation, Graz University of Technology, 2019. doi:10.13140/RG.2.2.31717.76004, Link
- S. Schrunner: Optimal Bayesian experimental design for crossover semiconductor lifetime studies, Master's Thesis, University of Klagenfurt, 2016. Link
- S. Schrunner: Bestimmung der First-Passage-Time Verteilung von Degradation-Path-Modellen, Bachelor's Thesis, University of Klagenfurt, 2014.
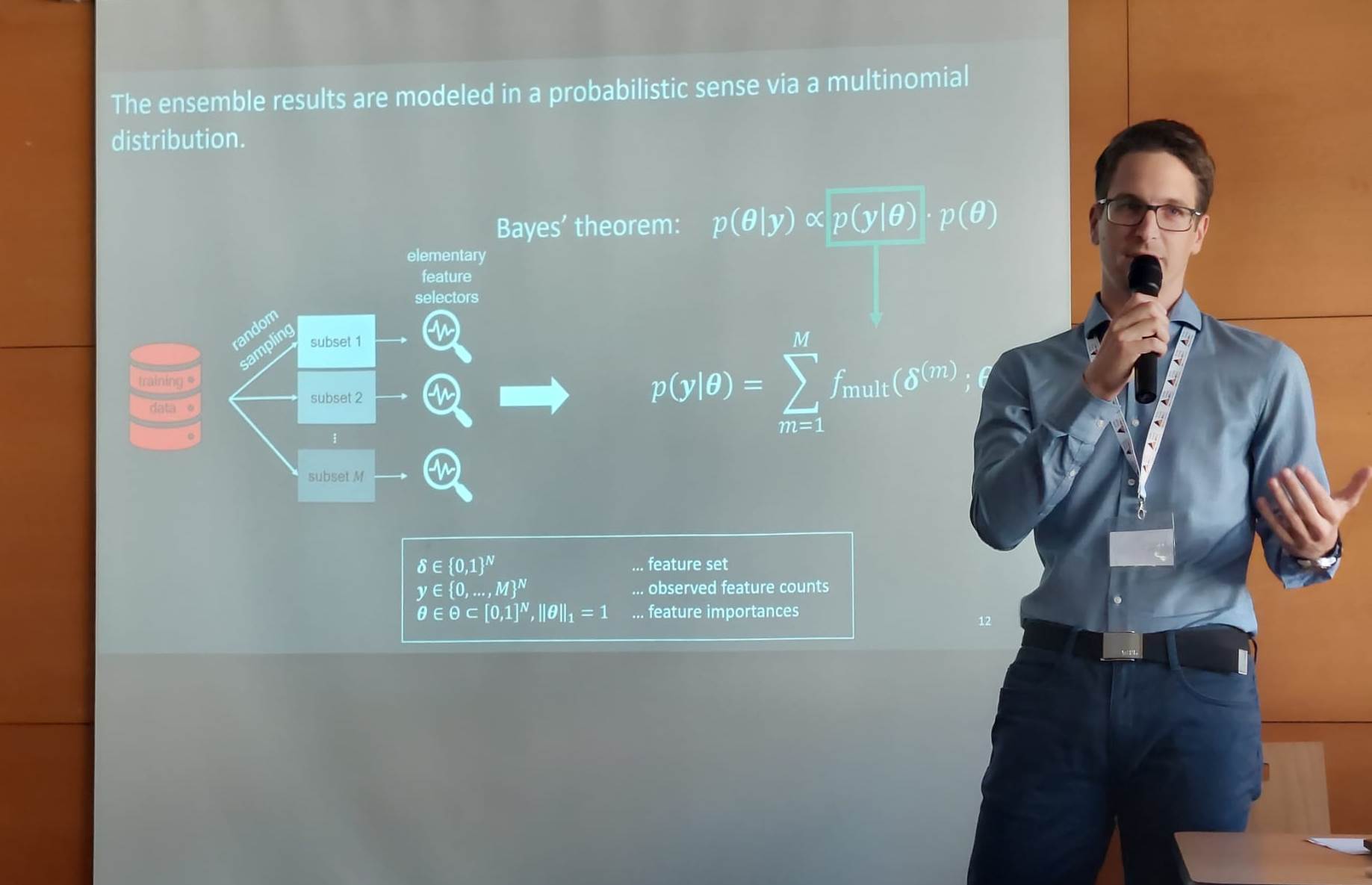
Teaching & supervision
Carinthia University of Applied Sciences
- Artificial Neural Networks and Deep Learning (I), spring 2025
- Artificial Neural Networks and Deep Learning (II), fall 2024
- Advanced Topics fall 2024, co-teaching with Prof. Jürgen Pilz
Norwegian University of Life Sciences
- DAT320 Sequential and time series data analysis, fall 2022 & 2023
- DAT121 Datavitenskap innføringsemne (introduction course for data scientists), fall 2021 & 2022, co-teaching with Prof. Kristin Tøndel and Prof. Hans Ekkehart Plesser
- INF221 Computer Science for Data Scientists, fall 2021, co-teaching with Assoc. Prof. Fadi Al Machot and Prof. Hans Ekkehart Plesser
Supervision
- N. R. F. Lizana and E. Risvik, "Missing Value Imputation and Survival Analysis for Treatment Outcome Prediction in High-Grade GEP NEN", Master's thesis, Norwegian University of Life Sciences, 2024 (co-supervisor)
- V. Molaug, "Prediction of Bus Dwell Time using Time Series Analysis and Machine Learning", Master's thesis, Norwegian University of Life Sciences, 2024 (co-supervisor)
- Z. R. H. Mustafa, "Exploring ensemble outlier detection methods on healthcare data for improved model predictions and cost optimization", Master's thesis, Norwegian University of Life Sciences, 2024 (co-supervisor)
- S. Rokhideh, "Treatment Outcome Prediction in Locally Advanced Cervical Cancer: A Machine Learning Approach using Feature Selection on Multi-Source Data", Master's thesis, Norwegian University of Life Sciences, 2023 (supervisor)
- E. D. Helland, "Tackling Lower-Resource Language Challenges: A Comparative Study of Norwegian Pre-Trained BERT Models and Traditional Approaches for Football Article Paragraph Classification", Master's thesis, Norwegian University of Life Sciences, 2023 (supervisor)
- N. Karki, "Extending the Repeated Elastic Net Technique for Multiclass Feature Selection: Performance Analysis and Comparison", Master's thesis, Norwegian University of Life Sciences, 2023 (co-supervisor)
- J. Szalas, "A Study of Statistical and Machine Learning Methods for Power Price Prediction Based on Filling Levels of Hydropower Reservoirs", Master's thesis, Norwegian University of Life Sciences, 2022 (co-supervisor)
- G. S. Gedde-Dahl, "Optimising maintenance operations in PV solar plants using data analysis for predictive maintenance", Master's thesis, Norwegian University of Life Sciences, 2022 (supervisor)
- A. Jenul, "Intensity Quantification of Process Patterns in Wafer Test Data", Master's thesis, University of Klagenfurt, 2019 (co-supervisor)
- M. Pleschberger, "Runtime Optimization for Automated Pattern Analysis", Master's thesis, University of Klagenfurt, 2018 (co-supervisor)
University of Klagenfurt
- 311.111 Übungen zu Analysis 2 (exercises in calculus), spring 2017
- 311.142 Tutorium zu Algebraische Strukturen (tutorial on algebra), fall 2015
- 311.158 Tutorium zu Lineare Algebra 2 (tutorial on linear algebra), spring 2015
- 311.154 Tutorium zu Lineare Algebra 1 (tutorial on linear algebra), fall 2014
Scientific software projects
RENT - Repeated Elastic Net Technique for Feature Selection
programming language: Python | year: 2021
GitHub |
UBayFSpy - User-Guided Bayesian Feature Selection in Python
programming language: Python | year: 2023
GitHub |
Block Importance Quantification in Artifical Neural Networks
programming language: Python | year: 2022
GitHub |
SlidingWindowReg - A Gaussian Sliding Windows Regression Model for Hydological Inference
programming language: R | year: 2023
(under development)
GitHub |
SSC-UC - A Semi-Supervised Classifier for Datasets with Unknown Classes
programming language: R | year: 2023
(under development)
GitHub |
About
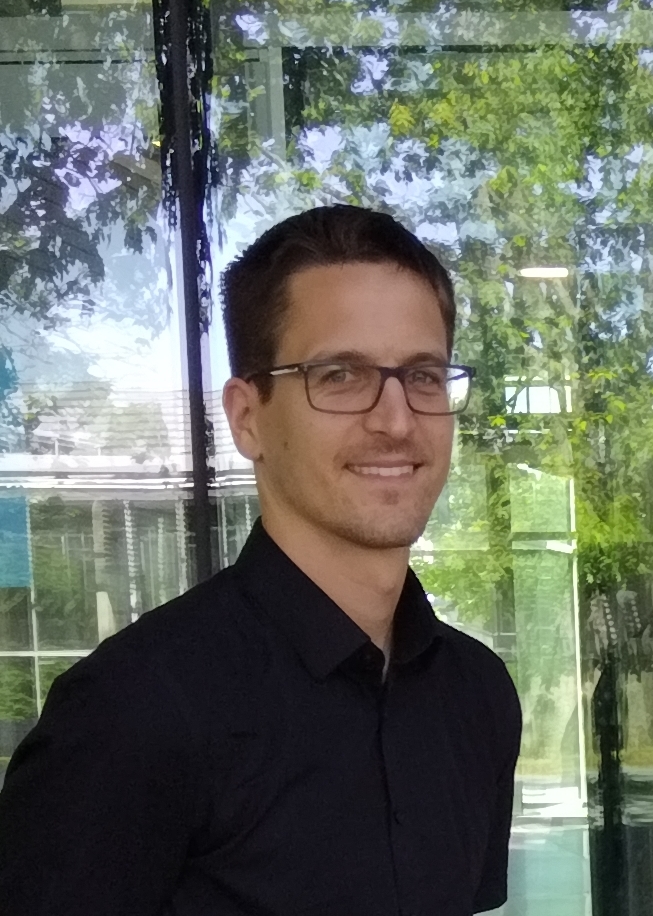
Conference and journal roles
- International Conference on Artificial Intelligence and Statistics (AISTATS) 2024, reviewer
- International Conference on Artificial Intelligence and Statistics (AISTATS) 2023, reviewer
- International Conference on Artificial Intelligence and Statistics (AISTATS) 2022, reviewer
- Knowledge-Based Systems (reviewer, 2023)
- Computer Methods and Programs in Biomedicine (reviewer, 2023)
- Robotics and Computer-Integrated Manufacturing (reviewer, 2023)
- Transactions on Semiconductor Manufacturing (reviewer, since 2019)
Skills & hobbies
- Programming: R, Python, Matlab, Java, C++
- Languages: German (native), English (professional), French (elementary), Norwegian (elementary)
- Professional memberships: IEEE (2015-2021), Austrian Statistical Society (since 2020)
- Hobbies: Scuba diving, swimming, hiking, skiing, traveling
- Voluntary experience: Active member of the Austrian Water Rescue Federation (ÖWR Faaker See)
Contact
Work address:
FH Kärnten gemeinnützige Gesellschaft mbH
(Carinthia University of Applied Sciences)
Europastrasse 4
9524 Villach, Austria
Website:
Email:
s.schrunner@fh-kaernten.at (work)
stefan.schrunner@protonmail.com (private)